In the field of cyberspace operations, most attack or exploitation operations are remote, i.e. they are carried out using technologies that allow the hostile actor not to be physically close to its target: access via VPN, a malicious email or link that installs an implant in the victim, a remote vulnerability that is successfully exploited, etc. But a small percentage of operations require a physical approach between the hostile actor and its target: these are proximity operations, also called Sneaker Operations or CACO (Close Access Cyberspace Operations).
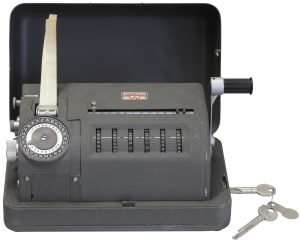
When not everything was connected to the Internet, proximity operations were almost the only way to access the target’s systems or information; to steal information you had to place a bug or a camera by sneaking into a building at night, modifying a supply chain or placing yourself in a building across the street from the target’s premises, to give a few examples. Some of the signals intelligence acquisition actions required this proximity, and this proximity obviously implied a significant risk of being neutralized, with all the implications that this neutralization can have. Some well-known examples of proximity operations for signals intelligence acquisition involve (allegedly) the French DGSE implanting bugs in the business seats of Air France flights between Paris and New York, the Soviets (allegedly) giving a Great Seal with an implant to the US ambassador to the USSR, or Germans and Americans (allegedly) manipulating Crypto AG cipher devices in Operation Rubicon.
[Read more…]